Advanced analytics: Boiling down the benefits of big data
In a data-rich world, could advanced analytical techniques be the key to higher performance in the automotive supply chain?
In this story...
Place an order with a major online retailer and you will probably be offered a range of recommended purchases. “Customers who bought this item also shopped for…” etc. Sometimes the articles on display will be bemusing, or even downright disturbing, but in many cases they will be genuinely useful.
Smart recommendations are one of the most common examples of a phenomenon that is reshaping the way many businesses run their operations. The principle is simple, but compelling: collect huge quantities of information on customer preferences, habits and behaviours, then slice it in clever ways to predict – and change – customer behaviour. This combination of ‘big data’ and analytics is also shaping things behind the scenes: knowing what you and your neighbours are likely to buy allows internet retailers to ship the right inventory to regional distribution centres, thereby cutting delivery times.
So, could these technologies transform the automotive supply chain? In some ways they have already begun to. “Big data has been in automotive for 20 years,” says Tim Clark, head of manufacturing solutions for the UK and Ireland at software company SAS. “The difference is that it is getting bigger, and the key question is how you get insights from that data and use those insights to make decisions.
“It’s not about storing data on huge servers, it’s about how you take those nuggets of information, look at patterns or clusters and use that data in forecasts,” he adds.
Matthias Wurst, programme manager for inbound logistics products at software company Inform, agrees that statistical methods have long been used in automotive – but now they are being extended to new functions, especially in predicting future patterns, starting with demand planning, anticipating how many parts need to be produced and delivered, and going right up to delivery punctuality. “Advanced analytics helps to increase the information you have about the status of the supply chain and gives you the chance to react before something happens in reality,” says Wurst.
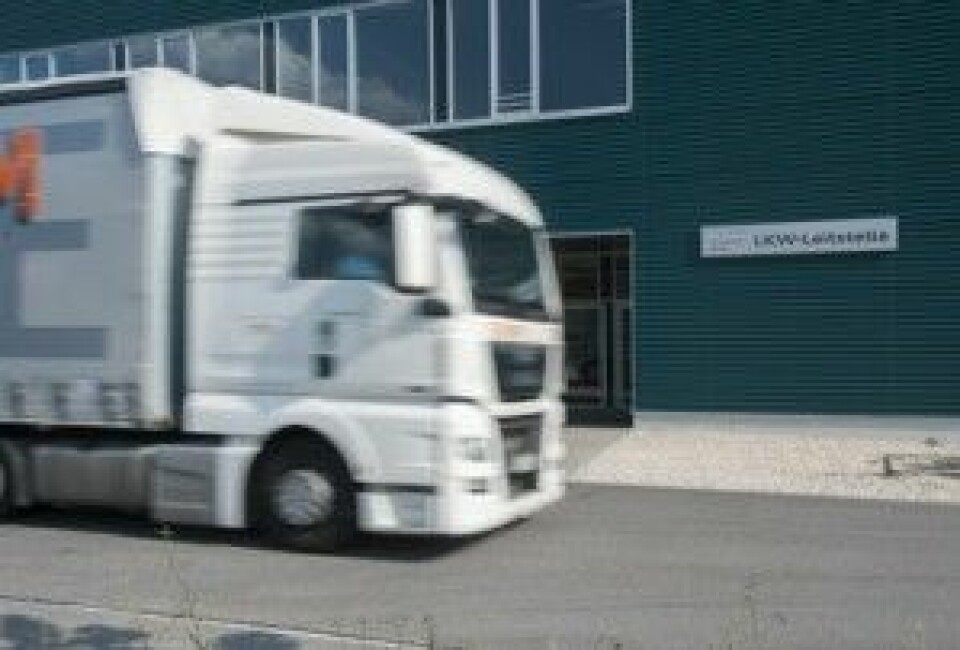
Some carmakers are using advanced analytics to predict whether a truck will be late, based on its position and current traffic conditions as well as historical timekeeping data
For example, Inform developed a slot-management system that has been adopted by carmakers to manage the scheduling of inbound parts deliveries to plants. It uses advanced analytics to predict if a truck will be late, based not only on its current position and the current traffic situation, but also on historical data, such as how often it has been late before.
In such systems, the richer the data available, the more accurate its predictions are likely to be. Inform already provides carriers with mobile apps that allow vehicles to share precise locations using GPS, [sta_anchor id="1"]but now the company is working on a pilot system that interacts directly with trucks’ on-board telematics systems. Such systems don’t just give the position of a vehicle, they can also provide information that might affect journey times, like the requirement for drivers to take a statutory break.
Changing behaviour, automatically The next logical step, which Inform is already working on, is a move from presenting relevant insights to users to making direct recommendations. “In the simple example of a delivery from a supplier that is often late due to traffic delays, the system will identify that the truck usually leaves the supplier plant two hours before the designated delivery slot, and it will suggest that the logistics chain planner asks the supplier to start leaving an hour earlier,” explains Wurst.
At enterprise software company Oracle, Dominic Regan, senior director for logistics applications in Europe, Middle East and Africa, thinks the ability of advanced analytical techniques to drive concrete action is what makes them so powerful. “What differentiates the latest approaches is that you are not just capturing and reporting data – you are using it to change supply chain execution.”
Regan thinks advanced analytics capabilities will lead to the development of “self-learning” supply chains. “You will see the development of systems that can pull in data from multiple sources, analyse that data, understand it and – within constraints – make decisions based upon it.” That would be a change in the role of front-line logistics planners and managers. “Analytics will be used less as a decision support tool and more as a decision validation system.”
"Advanced analytics helps to increase the information you have about the status of the supply chain and gives you the chance to react before something happens in reality." - Matthias Wurst, Inform
It’s an approach that logistics executives at carmakers such as BMW also foresee; its head of logistics, Jürgen Maidl, has described moving from a centrally driven supply chain to one that is ‘self-steering’ by way of autonomous vehicles and analytics.
Advanced analytics isn’t all about real-time data, notes Regan, but also spotting trends and conducting root cause analysis. He cites the use of historical delivery performance data to choose carriers for new logistics projects, or the use by carriers of dwell time at manufacturing plants to optimise their own [sta_anchor id="2"]schedules. “A carrier can look at the slots it is given by its customers and how good the customer is at honouring those slots,” he explains. “Are they predictable or do we need to build an allowance for more uncertainty into our schedules?”
Multiple data setsDHL has developed a supply chain risk management tool – Resilience360 – that makes extensive use of multiple data sets. The system grew out of an internal effort at the company to find new uses for its data on global transport footprints, explains Tobias Larsson, head of Reslience360. That data offered insights on how much ports, airports and other supply chain nodes were susceptible to disruption. The company has combined internal resources with other risk data from external sources to create its offering.
During sourcing and supply chain planning, for example, Resilience360 uses historical data to provide information on the exposure of particular locations to more than 20 different types of supply chain risk, from natural disasters to labour unrest, allowing companies to include security of supply alongside other considerations such as manufacturing cost and delivery lead times as they evaluate network options.
Using data supplied directly by customers and gathered from suppliers, DHL builds detailed models of supply networks in the system. These models show where supplier and customer sites are located and how parts and finished products are transported between them.
This approach can help industries such as automotive get to grips with risks in the lower tier supply chain, which companies often don’t fully understand. Multi-tiered supply networks can contain hidden “single points of failure”, for example; Larsson cites recent examples where many suppliers relied on the same single producer of specialist materials, creating widespread disruption when that supplier faced production problems. To address this issue, DHL has developed an online survey tool, called the R360 Transparency Portal, which collects data in an iterative manner from each tier of the customer’s supply network.
Once supply chains are up and running, the tool offers a near real-time monitoring function. Combining data from a variety of different feeds, including its internal transport management systems, as well as conventional and social media, DHL looks for information that could indicate disruptions. “Together with partners, we are developing a hierarchical taxonomy of thousands of search terms covering everything from fires to regulatory changes and financial issues at relevant companies,” explains Larsson. “Our listening tools constantly monitor various media sources for those terms, and the results are passed to a team of analysts who evaluate the information, take steps to confirm an incident and estimate its likely impact. The system then alerts our customers.”
The human link in the chain is essential, he notes, to qualify information before passing it to customers, and to ensure that alerts are cleared as soon as the disruptive event has passed.
“Not every piece of information we see can be qualified by our analysts,” Larsson admits. “But it is also important to look at trends in the unqualified data. One social media post about financial problems at a supplier or a pollution incident may not be relevant, but once you have, let’s say 50 separate reports, there is likely to be an issue.”

It was that sort of media monitoring that gave the first indication of problems at Hanjin Shipping, the Korean container line that went into receivership in August, he adds.
Combining customer shipment and bill of material data with the information on disruptions allows the system not only to alert customers to potential problems, but to show them exactly which products are likely to be affected. That makes it easier for DHL or its customers to take pre-emptive action to minimise the impact of delays.
Combining multiple data sources can reveal unexpected insights within companies. QuantumBlack, an analytics firm recently acquired by consultancy McKinsey & Company, says that by combining five years of data from 30 different sources, it could identify organisation and process improvement [sta_anchor id="3"]opportunities in new product development for an unnamed automotive company. QuantumBlack says its approach, which among other things looked at how individuals and teams with the company interacted by email and other means, found ways to cut time-to-market by 10% and costs by 11%.
Making advanced analytics workSeveral things need to be in place to make analytics effective tools for automotive logistics companies. One is the processing power to crunch data fast enough to make real-time decisions. Another is the right data, in an accessible form, which can be hard to come by.
“With advanced analytics you are looking at multiple different data sources,” says Tim Clark of SAS. “Not just the structured data, like sales ledgers and delivery invoicing, but also unstructured data like weather, order notes or social media information.”
Handling that diverse range of sources wasn’t a consideration for the designers of systems that many manufacturers use today. However, even structured data is becoming available in ever-larger quantities and more diverse forms, from smart machines on the production floor to cars themselves, which are beginning to store and communicate rich data on their own performance and reliability.
More modern software has been built with scalability and expandability in mind, but Dominic Regan warns that just adopting the latest doesn’t mean the integration challenge is solved. “We are streets ahead of where we were even ten years ago in terms flexibility and scalability but that doesn’t mean we are living in the age of plug and play,” he says. “Part of the challenge is that the evolution of technology means anyone can bring a device or system onto the market, and there is still little standardisation in the way he delivers data.” Data standardisation, he notes wryly, has been a constant theme of his career in supply chain technology spanning three decades.
"Our listening tools constantly monitor various media sources for [search] terms, and the results are passed to a team of analysts who evaluate the information, take steps to confirm an incident and estimate its likely impact." - Tobias Larsson, DHL
Any organisation hoping to wring insights out of big data sets needs people with the right skills to run their smart systems. It is likely to be a seller’s market for the foreseeable future, as logistics functions will compete for talent across other departments and with other industries. “If I was going to recommend a career option to anyone right now, I’d suggest something in knowledge science,” says Regan.
Then you have to get people to use the new tools as they become available. Wurst notes that while there is much interest in digitalisation among senior managers in automotive companies, the wider organisation often takes more convincing. “People still like to check the results of their logistics planning systems with a calculator. If the recommendation comes as the result of an advanced analytics approach, you can’t do that.”
Regan, however, thinks front-line personnel will be more willing to accept automated support in their work. “As long as they can see the value, people are willing to embrace new tools,” he reckons.
So, is the reward really the effort? Compared to other industries, says Wurst, automotive supply chains can reap big benefits from advanced analytics. “The supply chain is already highly optimised and every deviation in the process has a bigger impact on what happens in the factory.”
“Supply chains are complex and margins remain very tight in the automotive industry,” adds Tim Clark. “To succeed in that environment you need complete end-to-end visibility and a great deal of agility. The only way you achieve that is by truly understanding the data in your business and the markets you are approaching.”