Automotive Logistics reports on the Fraunhofer Institute’s research into autonomous warehouse decision-making and how it is working to deliver practical advantages from Industry 4.0 concepts
In this story...
Ranging from manufacturing engineering and applied materials research to process engineering and packaging, and from applied optics and precision engineering to machine tools and forming technology, each institute derives roughly two-thirds of its income from contract research, with government grants making up the balance.
Put another way, developments that start life in a Fraunhofer research centre have a knack of finding their way into German industrial best practice – and from there into business practice around the world. The MP3 music compression algorithm, for instance, was invented and patented by Fraunhofer, earning it over €100m ($112.5m) a year in royalties by the mid-2000s.
Such a track record should make it interesting for those in the automotive logistics industry to listen to what experts from Fraunhofer’s Dortmund-based Institute for Material Flow and Logistics (IML) have to say and are researching. As with the UK’s Cranfield University (with which it cooperates), IML’s academics and engineers combine academic rigour and research budgets to address everyday business challenges, but with a time horizon and freedom rarely found in the private sector outside noted ‘moonshot’ pioneers such as Google, Amazon and SpaceX.
[sta_anchor id="1"]4.0 reasons to look aheadRelated themes around data, connectivity and autonomy in production top IML’s agenda these days, explain Christian Schwede, head of the department of information logistics and decision support systems at Fraunhofer, and Dr Boris Otto, who is head of the Industrial Data Space initiative at Fraunhofer, as well as professor at Dortmund’s Technical University, where he is chairman of the Audi-Endowed chair of supply net order management.
Top of the list, explains Otto, is the Industrial Data Space initiative – a German-led attempt to underpin the emerging Industry 4.0 initiative (also German-inspired) with a secure data-sharing framework to support the exchange of digitised information up and down supply chains.
The second initiative, adds Schwede, is Smart Face, an attempt to envision and develop a paradigm for applying Industry 4.0 to vehicle manufacture, combining production and logistics in wholly new ways. This will be necessary, he stresses, because the next phase of industrial development will break down the traditional relationship between vehicle volumes and model variants and customisation. (‘Smart’, as it turns out, means simply ‘smart’; while ‘Face’ is an acronym for a lengthy German phrase roughly translated as ‘electric vehicles and lean production planning’.)
In particular, Schwede explains, Smart Face recognises that Industry 4.0, within the world of automotive manufacturing, will require a great deal more device- and equipment-level autonomous behaviour within the supply chain. That is where electric vehicles come in – AGVs and drones, certainly – but it also includes equipment within the supply chain of a more static nature: racks, for instance, that can order their supplies autonomously; bins that know their contents and can direct and control materials replenishment processes; containers that are able to organise routes and loads on their own; and so on.
“In an era of increased flexibility and greater variety, centralised planning breaks down – there’s too much human energy wasted in trying to predict what will happen and trying to then impose a flow on it,” argues Schwede. “A better policy is to have a system that is very transparent, and where, instead of trying to predict what will happen and then planning for it, the objective is to respond to actual events with logistics processes and capabilities that can react autonomously.”
Moreover, he adds, even Industry 4.0’s most ardent proponents concede that no real plan exists for the massive increase in data that supply chains will deliver. People talk glibly about Big Data and analytics, he points out, but at the level of plant and warehouse scheduling the need is for immediate decision-making and not more leisured insight creation. In other words, explains Schwede, there’s a real risk that Industry 4.0 will result in an actual decrease in decision-making transparency, as supply chains and supply chain planners become engulfed in data.
[sta_anchor id="2"]Left to their own, devices...However, autonomous decision-making – at a plant-floor and warehouse-floor level – helps resolve the problem: throw the data out to the devices that must make it happen and let them get on with delivering it.
Science fiction? Far from it, stresses Schwede, pointing to one of a number of real-world experiments carried out at the Fraunhofer IML to test such autonomous decision-making, especially in the context of cellular transport systems. In it, a swarm of 50 autonomous shuttles meets the requirements placed on a typical warehouse picking face or materials replenishment facility, deciding for themselves how to accomplish what must be done.
“We’ve had it running for five years now,” he explains. “Compared to the use of expensive fixed conveyors, it is much easier to make layout changes with shuttles. They can communicate with each other, and organise themselves. There is no central control system, and no lines embedded in the floor to guide them. Plus, it can be scaled very quickly: the swarm of shuttles can be added to, or subtracted from, very easily.”
What’s more, he adds, compared to traditional fixed material handling equipment, there are attractive cash flow advantages: businesses can start small, and then scale up as required, without needing to make a significant upfront investment. Plus, there’s nothing to prevent businesses renting their shuttles, scaling their numbers up and down as demand changes.
"Data does not equal knowledge. Industry 4.0 is an appealing idea but humans will want to know what is going on and how they can influence it. In our view simulations will become necessary in order to establish how the system will react under given circumstances and constraints." - Christian Schwede, Fraunhofer IML
“It’s not a ‘thought experiment’,” stresses Schwede. “It’s proven technology and it works. And now we’re working on a project to have shuttle swarms working in an assembly context, as opposed to simply picking. Because production is more complex than picking – the challenge isn’t in getting it to work, it’s in getting it to work efficiently.”
A further project, Schwede adds, is exploring the practicality of low-cost AGVs – dubbed ‘locatives’ – intended to be cheaply deployed, installed using printed barcodes and adhesive tape for lane guidance, and capable of carrying high loads in comparison to their own weight. In the trials currently taking place at the Dortmund IML, for instance, locatives weighing just 8kg are capable of carrying 12kg, which can be a useful load in many aspects of automotive parts handling.
“The whole emphasis is on low cost; simple devices that are low-cost to buy and low-cost to install in your production environment,” he stresses. “The idea is that companies should be able to buy standard locatives from a catalogue and then install them themselves.”
Similarly, another project is exploring the use of autonomous drones within production and warehouse sites – including vehicle compounds. Partnering with the University of Bonn, the project is exploring the practicality of using autonomous drones for inventory checking, initially at night, when facilities are unmanned.
[sta_anchor id="3"]Still another project – the InBin – aims to develop intelligent bins, capable of not only storing components, but intelligently making decisions about reordering, sequencing, traceability, and monitoring the environmental conditions experienced by those components. Equipped with a 32-bit processor and 512K of memory, the InBin has a small ‘e-Paper’ screen for displaying status information and can communicate with mobile tablet computers such as iPads and Android devices. It is also self-powered, capable of running off the ambient light its photovoltaic receptors pick up, even in a typical warehouse environment.
While such technology certainly has many advantages, autonomous behaviour on this scale has a downside: it will not necessarily be more transparent, says Schwede.
“Data does not equal knowledge,” he observes. “Industry 4.0 is an appealing idea but humans will want to know what is going on and how they can influence it. In our view, simulations will become necessary, in order to establish how the system will react under given circumstances and constraints.”
On the other hand, he adds, autonomous decision-making at device-level has the potential to be directed far more precisely and far more consistently than at a human level.
In effect, he argues, a production or logistics environment populated by autonomous devices is a production or logistics environment that has ‘levers’ that can be directly influenced by management. Devices, in other words, can be told to focus on due-date performance, or efficiency, or low inventory; device by device, that focus will be obeyed, instantly and without question.
[sta_anchor id="4"]Giving safe space for dataAll of which begs the question of how all these devices are to communicate, both with each other, and with the enterprise systems that operate at a human and system-to-system level. Nor is such communication restricted to inside the enterprise: when an autonomous bin decides that it needs replenishing, for instance, that requirement (and the response to that requirement) needs to travel along the supply chain.
It’s here, says Schwede’s colleague Boris Otto, that IML’s work in respect of the Industrial Data Space initiative comes into play.
The starting point is a recognition that while Industry 4.0 and the Internet of Things can provide unparalleled visibility and granularity of information in respect of supply chains, there is a need for a secure and consistent way of sharing that data. Secure, explains Otto, because much of it may be sensitive; and consistent because data that is open to interpretation – and misinterpretation – is of limited value.
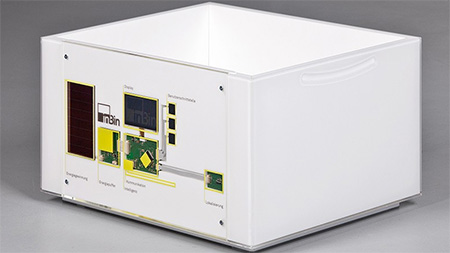
“It’s vital that everyone in the supply chain has the same understanding of what the data means and what it is telling them. Even terms such as ‘estimated time of arrival’ may mean different things to a car plant and a logistics service provider; there need to be agreed definitions and tolerances.”
To this extent, he adds, the barriers that the Industrial Data Space initiative must overcome go beyond the purely technical, and embrace organisational and semantic challenges as well. Hence, he points out, the need for a broad constituency of interests to come together to reach a consensus.
In the case of the automotive industry, he argues, the challenges are made much easier because the industry’s logistics and material handling processes are well known and well understood, and most of the players involved have long-standing relationships.
“It’s not like other industries. We just need to get on and do it,” urges Otto. “And what helps by way of a tailwind is that no one player has all the data. The various partners involved will have to come together to reach a consensus.”
That said, the role of the Fraunhofer IML is to accelerate and support that process, a mission encouraged and funded by Germany’s Federal Ministry of Education and Research.
“There was a view that digitisation was going to happen and that Germany shouldn’t wait for the large United States-based IT vendors to come up with a solution; we should, as an industry, take the future into our own hands,” Otto explains.
Accordingly, a meeting of a dozen or so large German carmakers and tier suppliers was convened at the end of 2014, with the Fraunhofer IML submitting a proposal on how such an initiative might be established. Government funding was provided for an initial three years, contingent upon Fraunhofer IML providing evidence that the initiative might work, and that industrial partners would actually join it.
This initiative in turn led to the formal creation of the Industrial Data Space Chartered Association in January 2016 and a related programme of research at the IML to further explore digitisation.
However, Otto is keen to refute any impression that the initiative is a German-only affair, emphasising that its goals remain focused on solid benefits and a broad pan-national consensus.
“Coupled to perceptions of German over-engineering, there’s sometimes a sense that Industry 4.0 is an end in itself. Instead, there are very definite gains for the industry,” he stresses.
“As for nationalities, the current 30 or so members come from six or seven countries. It’s definitely not a ‘German-only’ initiative and a number of members have said that if it became a German-only project they wouldn’t want to take part.”
Even so, the initiative’s work is still only in its early stages of research and development, with the Fraunhofer IML working on a framework reference architecture model and the development of use cases. These are due to be finalised by October 2018. Until then, while we are likely to hear more about the Industrial Data Space project, it is – for once literally – a case of ‘watch this space’.
"It’s vital that everyone in the supply chain has the same understanding of what the data means and what it is telling them. Even terms such as ‘estimated time of arrival’ may mean different things to a car plant and a logistics service provider." - Boris Otto, Fraunhofer IML